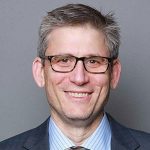
By Jeffrey Feinstein, PhD, vice president, global analytic strategy, LexisNexis Risk Solutions, and Alanna Shuh, director, fraud and identity, LexisNexis Risk Solutions
Fraud is an escalating challenge in Canada, particularly for financial institutions. The 2023 LexisNexis Risk Solutions True Cost of Fraud study indicates that Canadian financial services fraud executives reported an almost 30 percent year-over-year increase in monthly fraud attempts and the average number of successful monthly fraud attempts more than doubled.
This begs the question, what tools can detect and prevent rising and increasingly sophisticated fraud attacks? More specifically, how can advanced technologies such as Artificial Intelligence (AI) support fraud prevention efforts, regardless of channel?
To effectively thwart bad actors, it is important to understand the elements that define a trusted user versus a fraudster. Obtaining a 360-degree view of a consumer from a physical, digital and behavioral perspective then uncovering new anomalies indicative of illicit activity is paramount as fraudsters continually devise new ways to appear legitimate, evading one-dimensional fraud controls.
Layering defenses that are stacked in progressively greater levels of friction at higher risk consumer touchpoints is critical given the heightened level of attacks and demand for exceptional customer experiences. Today, several tools help identify anomalous characteristics or behavior, across the customer journey, which not only employ AI but also in many cases require minimal user interaction.
There are many forms of AI, some of which are already well-established in the financial services sector. AI encompasses, “Machine-based systems which infer solutions to set tasks and have a degree of autonomy,” which include traditional computer-derived models as well as other subcategories. Machine learning (ML) can be defined as a “subcategory of AI [that] uses algorithms to automatically learn insights and recognize patterns from data, applying that learning to make increasingly better choices.”
Extractive AI and ML, a commonly used branch of AI, lift relevant data points from the data on which it has been trained which can be used to perform unsupervised anomaly detection and predict potential fraud, to name a couple of examples. Companies frequently leverage this form of AI today and deploy it across consumer touchpoints in various forms.
While generative AI is the hot topic du jour, there are existing variations of AI that are both available and established that are enormously helpful today for fraud prevention. Below are some common examples of AI and ML used for fraud detection and prevention in both digital and physical channels. Of note, organizations seeking to use AI should ensure that solution vendors abide by responsible AI principles to ensure model explainability, accuracy, privacy and other core tenants of good AI.
Digital Channels (Online and Mobile Applications)
Anomaly detection is identifying events or data points that deviate from an expected distribution of behavior. Essentially, anomaly detection spots data elements or behaviors that differ from ‘normal’. Stealing or creating identities and then acting on them is incredibly challenging. Elements often appear different, raising questions about whether the current behavior matches past transactions of the identity. Additionally, synthetic identities can be detected by comparing their emergence patterns with those of other low-risk identities. In a sense, bad actors try to simulate legitimate behavior and anomaly detection is designed to identify patterns that do not match what is expected.
In practice, in digital workflows and assessing numerous elements – such as device attributes, digital identity, behavior, activity, location and more – to identify inconsistencies can inform whether to permit an action, require additional authentication or decline a user. AI and ML are frequently at the core of risk-decisioning engines, behavioral biometrics, document authentication and email risk intelligence products, which effectively help determine good users from bad.
Taking behavioral biometrics as an example, technologies verifying behavior can assess keyboard signals, mouse movements, touchscreen interactions and mobile sensors to create an anonymized profile associated with the user. It is impossible to perfectly mimic how an individual interacts with their device, which is why models assessing behavior can be so powerful in spotting a potential account takeover or bot.
Case studies have revealed that utilizing behavioral biometrics can capture 63 percent of fraud at a three percent intervention rate and fraud capture is even greater when combining behavioral biometrics with additional fraud risk analytics capabilities. Behavioral biometric models combined with digital identity intelligence can also support identifying potential victims of a scam mid-flight.
When a scam artist actively coaches an individual, the victim’s behavior often changes to reflect that they are following instructions or acting under duress. Behavioral biometrics is one example of AI embedded tools combatting fraud, however combining solutions assessing multiple characteristics in a stacked approach has proven to be most effective in reducing both successful fraud attempts and the cost to address fraud.
Physical (Branch or Store)
AI and ML can be used in physical retail locations for document authentication. Leading document authentication software performs dozens of tests per ID scan to detect fake documents and include ML models trained on thousands of ID documents. Key examples of AI usage in physical use cases include ML models that detect digital manipulation like photo substitution, text tampering and printing of screen capture or photocopies.
Fraudsters are known to prey on retail stores to open new accounts, perform high-value transfers and other high-risk activities, which is partially responsible for 25% of fraud losses occurring at retail branches. Fake IDs now include high-resolution, high-quality replicas of legitimate IDs, making manual review nonviable.
Organizations that have implemented ID scanners or tablets with document authentication have been known to spot fraudsters within weeks, sometimes hours, after implementation and have stopped high-dollar criminal activity such as attempted title fraud. Gaining strong authentication results, supplemented with details about AI attack potential (e.g., photo substitution), empowers branch managers to confidently reject fraud attacks.
Summary
Regardless of channel, identifying abnormal behavior and characteristics is essential in identifying and preventing fraud. Many solutions today use technologies such as AI to understand a good user so that organizations can easily spot and stop suspicious activity.
With AI- and ML-powered insights, organizations can better detect increasingly challenging schemes such as scams, stolen identities, synthetic identities and other forms of fraud. Explainable AI provides organizations with the capabilities to manage fraud risks while limiting false positives that could impact consumers’ experiences. Beyond detecting fraud, these technologies can also be helpful in reducing manual efforts associated with reviewing and responding to fraud alerts, optimizing work queues and prioritizing risks.
For organizations seeking to better utilize these capabilities to their advantage, selecting vendors that abide by responsible AI practices is imperative. In the future, new tools such as generative AI may also have a place in supporting fraud prevention efforts, however model explainability and accuracy will be necessary for generative AI’s future success in fraud and financial crime prevention purposes.
LexisNexis Risk Solutions harnesses the power of data and advanced analytics to provide insights that help businesses and governmental entities reduce risk and improve decisions. LexisNexis Risk Solutions follows the Responsible Artificial Intelligence Principles at RELX.